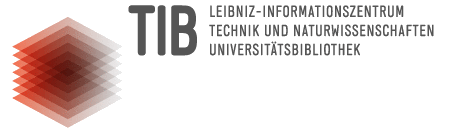
Sehr geehrter Kunde,
Es ist ein interner Fehler aufgetreten. Bitte entschuldigen Sie die Unannehmlichkeiten.
Der Fehler wurde von uns registriert und wir versuchen ihn schnellstmöglich zu korrigieren.
Bei Fragen wenden Sie sich bitte an den Webmaster